
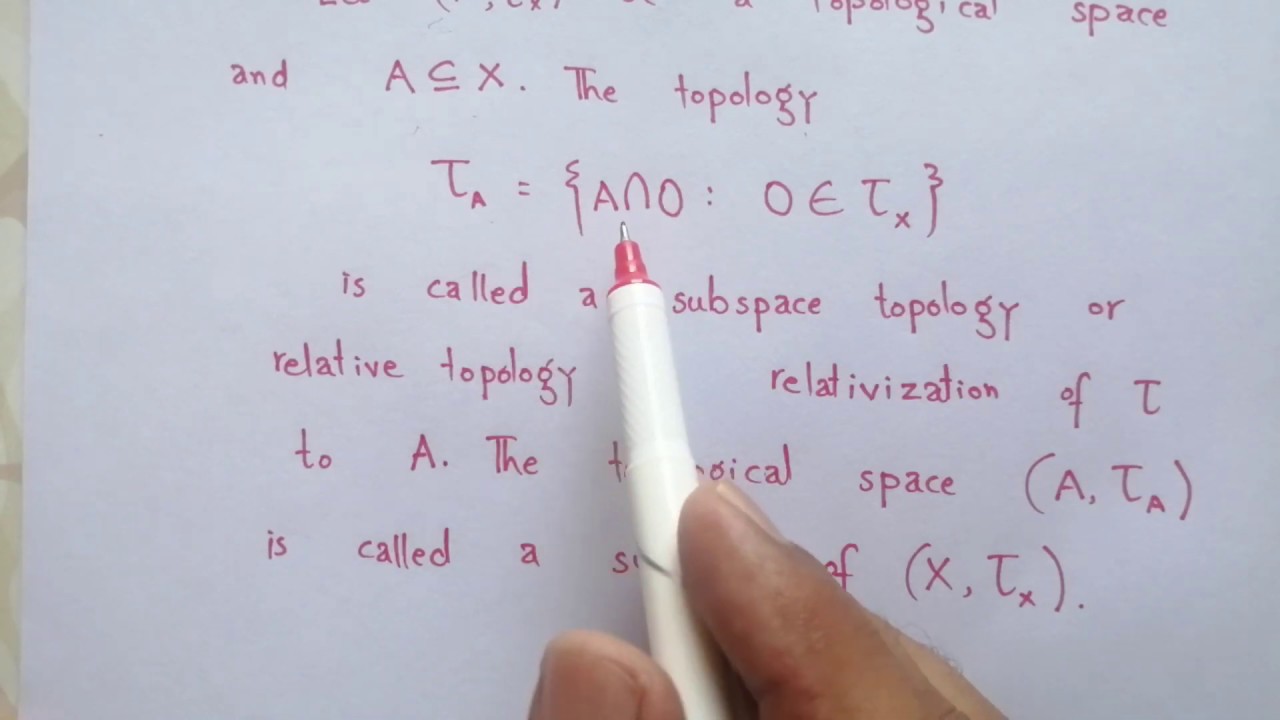
Error = ‖ y - Xθ ‖ This is the distance between y to the point Xθ which lies in the columns space of X.
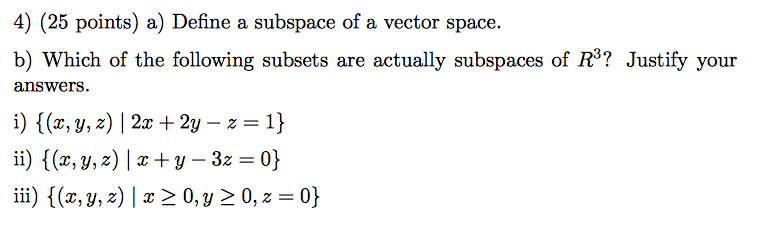

y will be outside of the column space C(X). In other words, y won’t be a combination of columns of X. So we expect that there will be no exact solution. The number of samples (3 in this example, or any m) usually will be much greater than the number of features (2, or any n). Think of X as a design matrix for which the number of samples is 3, the number of features is 2. Going back to eq.(a), X is a 3 by 2 matrix and θ is 1 by 2 matrix (no longer a scalar). Searching for θ that minimizes Error is the same as LOCATING the point Xθ as close as possible in the column space than any other point in the C(X). If we allow singular matrices, or rectangular matrices of any shape, then C(X) will be somewhere between the zero space and R^n. Any n by n matrix that is non-singular will have R^n as its columns space. Two 7-D vectors and will still span the plane.ģ. Just because they are 3-D vectors, they don’t span the 3-D plane, you need 3 vectors to span 3-D space. Why do and span the plane? They are 3-D vectors.īecause they are 2 vectors 😜. Therefore, “y lies in the column space” means the error of the linear regression is zero, which is never the case in real life.Ģ. θ is the coefficient that we are trying to fit. y is a target variable (the housing price). The number of rows in X is the number of training examples. X is a feature matrix or input variables (# of bedrooms, square feet, location, etc). Xθ = y can be solved only when y lies in the plane that is spanned by the two column vectors, the combination of the columns of X. It consists of every combination of the columns and satisfies the rule (i) and (ii). The spanned plane C(X) is not just a subset of R³. However, notice, if y lies off the plane C(X), then it is not the combination of the two columns. The column space C(X): A 2-D plane spanned in 3-D space. Hence, the smallest subspace containing the first quadrant is the whole R² space. However, now the rule (i) is violated, since adding + will result in, which is not in either quadrant. Every multiple cx will stay in this subset. If we include the third quadrant along with the first, scalar multiplication is all right. Now, cx = is in the third quadrant, not the first. How about the rule (ii)? Let’s pick any scalar c = -3 and the vector x = in the first quadrant. If we add any two vectors a (a1>0, a2>0) and b (b1>0, b2>0) in the first quadrant, a + b will be in the subspace. The first quadrant of the x-y plane: Is it a subspace? In order to solidify our understanding, let’s try to answer this question: Understanding the concept by only reading the definition doesn’t really work for me. (ii) If we multiply any vector x in the subspace by any scalar c, cx is in the subspace as well. (i) If any two vectors x and y are in the subspace, x + y is in the subspace as well. Definition of Subspace: A subspace of a vector space is a subset that satisfies the requirements for a vector space - Linear combinations stay in the subspace. These two operations keep the output within the subspace always. A subspace is a subset that is “closed” under addition and scalar multiplication, which is basically closed under linear combinations. Then if any two of the following statements is true, the third must also be true:įor example, if V is a plane, then any two noncollinear vectors in V form a basis.When people say ‘Vector Space’, ‘Column Space’, ‘Subspace’, etc., what do they mean by ‘Space’? A basis of V is a set of vectors in a subspace V. This is the idea behind the notion of a basis. There are infinitely many choices of spanning sets for a nonzero subspace to avoid reduncancy, usually it is most convenient to choose a spanning set with the minimal number of vectors in it. Essential vocabulary words: basis, dimension.Īs we discussed in Section 2.6, a subspace is the same as a span, except we do not have a set of spanning vectors in mind.Picture: basis of a subspace of R 2 or R 3.Recipes: basis for a column space, basis for a null space, basis of a span.Understand the definition of a basis of a subspace.Section 2.7 Basis and Dimension ¶ Objectives Hints and Solutions to Selected Exercises.3 Linear Transformations and Matrix Algebra
